AI and automation are key technologies that can address the manpower shortage and skills gap.
AI is driving the Industry 4.0 revolution across both discrete manufacturing as well as process industry. One of the key technologies behind this revolution is advanced analytics powered by machine learning, also referred to as Predictive Analytics. According to a Global CEO Survey conducted across manufacturing companies by Deloitte, executives ranked Predictive Analytics as their highest priority technology vital to their companies’ future competitiveness. Today, there are many ways for Smart Manufacturing to leverage predictive analytics from smart maintenance (anomaly detection) real-time quality monitoring to the production process, and supply chain optimization. However, the digital transformation journey in manufacturing is riddled with potholes. CIOs and DX leaders should think through several challenges they will likely face and must have mitigation strategies in place in order to move from AI proof of concepts to AI in production at scale:
- Legacy Machinery, Disconnected Systems: The production equipment market is fragmented and consists of several regional and global players. In the manufacturing machinery market, lack of system interoperability is a common issue. Vendors offer a plethora of technologies and components that are incompatible with other systems. Industrial IoT lacks standards and frameworks. Customers still worry about connectivity, how can they connect legacy machines and often start at the fundamental level – the sensors or convertors they need to install. An ecosystem of players that can offer compatible components that use standard rules and frameworks to connect to ERP, MES, and PLC/SCADA systems is the right way to approach this problem. OPA UA is becoming the key protocol for Industry 4.0 communication and data modeling.
- Inability to Access & Leverage Data – The production floor, heavy manufacturing environment in particular is characterized by extreme, harsh operating conditions. Fluctuating ambient temperature, pressure, dust, and vibrations are challenging conditions and wide variations can add noise to data leading to data inaccuracies. The manufacturing site may also be at a remote location, bringing additional complexities in terms of data storage. The IT team may also not want to send data to the cloud due to security concerns, requiring on-premises solutions. Any analytics application will need access to data from a computerized maintenance management system(CMMS) or process historians and may require database connectors, or custom scripts. In order to execute any advanced analytics or IoT initiative, strong collaboration between operations technology (OT) and information technology (IT) is required.
- Demand for Real-Time Response – Condition monitoring is a common use case in what’s known as “Industry 4.0” where real-time data from an asset is analyzed using streaming analytics to ensure optimal performance. There are many applications in manufacturing that are sensitive to latencies such as predictive maintenance or predictive quality and require an ultra-fast response. For these applications, the system cannot wait for the round trip journey to the cloud to perform data processing and get actionable insights. The decision has to be made in real-time, acted upon immediately in few milliseconds. That’s where edge computing can make a difference. It becomes more efficient to process data locally near the source of data for faster response. Real-time decision making and local control systems need edge-based computing. The ability to deploy predictive models on the edge devices such as machines, local gateway, or server is absolutely critical to enable smart manufacturing applications.
- Multi-Disciplinary Tech Skills & AI Readiness – AI projects require multi-disciplinary teams with expertise in data management, algorithms, and machine learning. Most OT experts are not trained in building predictive models or using advanced analytics powered by AI and ML. Instead, they rely on teams of data scientists and advanced analytics to assist them in using the latest technologies in operations. How can manufacturers hire AI and ML talent when it is in such short supply? The perception that the manufacturing industry is risk-averse, not sexy or cool makes it even harder to attract AI talent. DX leaders should look at automated ML tools that OT experts can leverage to build predictive models without requiring data scientists. The new AutoML platforms automate up to 100 percent of the AI/ML development workflow, using an AI-based engine to automatically discover meaningful patterns and build ML-ready feature tables from relational, operational, temporal, geo-locational, and text data
- Bias Against AI & Trust – In the manufacturing world, reliability is absolutely critical, and high accuracy is required since the stakes are huge. A research conducted by Aberdeen Research highlighted that 82% of companies experience downtime and the cost of unplanned downtime can be as high as $280,000 per hour. To avoid expensive downtime, OT experts trust their domain experience, intuition, and gut feeling over any algorithm. Black-box modeling approach where the operator cannot understand how the algorithm selected parameters and what features led to a prediction makes matters worse. Leaders should utilize white-box modeling that offers a clear explanation and easy interpretation. Just as the manufacturing world embraced robots and cobots, the industry needs to embrace AI and ML. The manufacturing industry is projected to face a severe shortage of manpower due to the looming retirement of skilled employees. AI and Automation are key technologies that can address this gap while boosting operational efficiency, quality, and productivity.
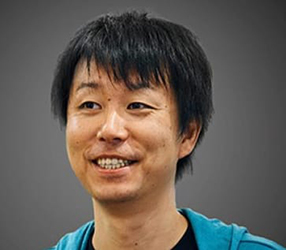
Ryohei Fujimaki
About Ryohei Fujimaki, Ph.D.
Ryohei Fujimaki is the Founder & CEO of dotData, a spin-off of NEC Corporation and the first company focused on delivering full-cycle data science automation for the enterprise. Dr. Fujimaki is a world-renowned data scientist and was the youngest research fellow appointed in the 119-year history of NEC.